From Idea to Reality: AI-Powered Novel Generation with v5.2
Project Genesis
Unleashing Creativity: My Journey into AI-Generated Novels
From Idea to Implementation
AI 小说创作助手 (AI Novel Writing Assistant) 项目开发历程
1. 初步研究与规划
2. 技术决策及其理由
3. 考虑的替代方案
4. 关键洞察
总结
Under the Hood
Technical Deep-Dive: AI Novel Writing Assistant
1. Architecture Decisions
-
Modular Design: The application is structured into distinct modules, each responsible for specific functionalities such as text generation, prompt management, and user interface. This modularity allows for easy updates and integration of new features or AI models.
-
API-Driven Architecture: The application leverages APIs to connect with various AI models, enabling flexibility in choosing the underlying technology. This design choice allows users to switch between different AI models (e.g., ChatGPT, DeepSeek) without altering the core application logic.
-
User-Centric Interface: The interface is designed to be intuitive, with features like drag-and-drop mind mapping and context-sensitive right-click menus for text optimization. This focus on user experience enhances productivity and makes the tool accessible to both novice and experienced writers.
-
Data Management: The application includes a robust data management system that supports local storage, configuration import/export, and automatic saving. This ensures that users can work seamlessly without losing progress.
2. Key Technologies Used
-
Python: The primary programming language used for developing the application. Python’s simplicity and extensive libraries make it ideal for rapid development and integration with AI models.
-
Flask: A lightweight web framework used to create the web server that hosts the application. Flask allows for easy routing and handling of HTTP requests, making it suitable for building RESTful APIs.
-
JavaScript and HTML/CSS: Used for the front-end interface, enabling dynamic interactions and a responsive design. Libraries like jQuery may be utilized for DOM manipulation and AJAX calls.
-
AI Models: The application supports multiple AI models, including ChatGPT, DeepSeek, and others. These models are accessed via REST APIs, allowing for text generation and optimization based on user input.
-
Mind Mapping Libraries: JavaScript libraries for creating interactive mind maps, enabling users to visually organize their story structure and chapters.
3. Interesting Implementation Details
-
Prompt Management System: The application features a sophisticated prompt management system that allows users to create, modify, and store prompts for different writing contexts (e.g., outlines, chapters, content). This system supports variable placeholders (e.g.,
${characters}
,${plot}
) that can be dynamically replaced during text generation. -
Right-Click Optimization Menu: Each text section (outline, chapter, content) has a context-sensitive right-click menu that provides optimization options. For example, users can select a paragraph and choose to “Enhance Emotion” or “Add Details,” triggering AI-driven suggestions for improvement.
-
AI Iteration Feature: The application includes an AI self-iteration feature that allows users to refine their text based on AI-generated feedback. This feature uses a scoring system to evaluate the quality of the text and suggests improvements, enhancing the overall writing process.
-
Customizable Themes: Users can switch between light and dark themes, as well as customize colors to suit their preferences. This feature improves usability and comfort during long writing sessions.
4. Technical Challenges Overcome
-
Integration of Multiple AI Models: One of the primary challenges was integrating various AI models while maintaining a consistent interface. This was overcome by creating a unified API layer that abstracts the differences between models, allowing users to switch models seamlessly.
-
Performance Optimization: As the application handles large text inputs and complex AI interactions, performance optimization was crucial. Techniques such as lazy loading of components, efficient state management, and minimizing API calls were implemented to enhance responsiveness.
-
User Data Management: Ensuring that user data is securely stored and easily retrievable posed a challenge. The application uses local storage for quick access and implements a backup system to prevent data loss.
-
Dynamic UI Updates: Implementing a dynamic user interface that responds to user actions (e.g., generating text, updating prompts) required careful management of state and event handling. This was achieved using JavaScript frameworks and libraries that facilitate real-time updates.
Example Code Concepts
import requests
def generate_text(prompt, model='chatgpt'):
api_url = f"http://api.example.com/generate/{model}"
response = requests.post(api_url, json={'prompt': prompt})
return response.json().get('text', '')
def replace_variables(prompt, context):
for key, value in context.items():
prompt = prompt.replace(f"${{{key}}}", value)
return prompt
## Lessons from the Trenches
### Key Technical Lessons Learned
1. **Modular Design**: The project benefits from a modular architecture, allowing for easy integration of different AI models. This design facilitates the addition of new features and models without disrupting existing functionality.
2. **Prompt Engineering**: The importance of crafting effective prompts cannot be overstated. The system's performance heavily relies on the quality of the prompts used for generating content. A well-structured prompt can significantly enhance the output quality.
3. **User Experience (UX)**: Focusing on user experience is crucial. Features like drag-and-drop mind mapping and context-sensitive right-click menus improve usability and make the tool more intuitive for writers.
4. **Data Management**: Implementing a robust data management system is essential for handling user-generated content and configurations. This includes features for saving, loading, and exporting user data seamlessly.
5. **Feedback Loop**: Incorporating user feedback into the development process has proven invaluable. Regular updates based on user suggestions help in refining features and fixing bugs.
### What Worked Well
1. **AI Integration**: The ability to switch between multiple AI models (e.g., ChatGPT, DeepSeek v3) has been a strong point, allowing users to choose the model that best fits their needs.
2. **Efficiency Boost**: The introduction of features like the shift+L shortcut for quick input and the AI-assisted content generation has significantly increased writing efficiency for users.
3. **Comprehensive Documentation**: Providing detailed documentation and usage tutorials has helped users understand the tool better and utilize its features effectively.
4. **Community Engagement**: Actively engaging with the user community through feedback channels has fostered a sense of ownership and loyalty among users.
### What You'd Do Differently
1. **Testing and QA**: Implement a more rigorous testing and quality assurance process before releasing new features. This could help catch bugs and usability issues earlier in the development cycle.
2. **Performance Optimization**: Focus on optimizing the performance of the application, especially when handling large texts or complex prompts. This could involve refining the backend processes or improving the efficiency of API calls.
3. **User Onboarding**: Enhance the onboarding experience for new users. A guided tutorial or interactive walkthrough could help users familiarize themselves with the tool's features more quickly.
4. **Scalability**: Plan for scalability from the outset. As user numbers grow, ensuring that the application can handle increased load without performance degradation is crucial.
### Advice for Others
1. **Start Small, Iterate Fast**: Begin with a minimum viable product (MVP) and iterate based on user feedback. This approach allows for rapid development and adaptation to user needs.
2. **Focus on Core Features**: Identify and prioritize core features that provide the most value to users. Avoid feature bloat by ensuring that each addition enhances the user experience.
3. **Engage with Users**: Maintain open lines of communication with your user base. Regularly solicit feedback and be responsive to their needs and suggestions.
4. **Invest in Documentation**: Comprehensive documentation is essential for user adoption. Ensure that users have access to clear, concise guides and tutorials.
5. **Stay Updated**: Keep abreast of advancements in AI and writing technologies. Regularly update your tool to incorporate new features and improvements that can enhance user experience.
By following these lessons and advice, developers can create more effective and user-friendly applications that meet the needs of their target audience.
## What's Next?
## Conclusion: The Future of AI Novel Writing Assistant
As we stand at the forefront of the AI-driven literary revolution, the AI Novel Writing Assistant project has made significant strides since its inception. Currently, we have successfully empowered hundreds of studios and individual authors to rapidly and efficiently generate novels, achieving an impressive 20-fold increase in writing productivity through our advanced AI technology and comprehensive prompt management features. With the recent upgrade to version 5.2, we have introduced a plethora of new functionalities, including mind map construction, self-optimizing novel capabilities, and a robust writing knowledge base.
Looking ahead, our development plans are ambitious. We aim to enhance the user experience further by integrating more AI models, expanding our prompt libraries, and refining our intelligent optimization tools. Our goal is to create an even more intuitive interface that allows writers to focus on their creativity while the AI handles the intricacies of structure and style. We also plan to explore collaborative features that enable writers to work together seamlessly, sharing insights and ideas in real-time.
We invite contributors from all backgrounds—writers, developers, and AI enthusiasts—to join us on this exciting journey. Your insights, feedback, and creative input are invaluable as we continue to refine and expand this project. Whether you want to contribute code, share your writing experiences, or help us test new features, your participation will help shape the future of AI-assisted storytelling.
Reflecting on this side project journey, it has been a remarkable experience filled with learning and innovation. We have witnessed firsthand how AI can transform the writing process, making it more accessible and enjoyable for everyone. As we continue to push the boundaries of what is possible in novel writing, we remain committed to fostering a community that celebrates creativity and collaboration. Together, we can redefine the landscape of storytelling and empower a new generation of writers to bring their visions to life.
Join us as we embark on this exciting adventure, and let’s create the future of literature together!
## Project Development Analytics
### timeline gant
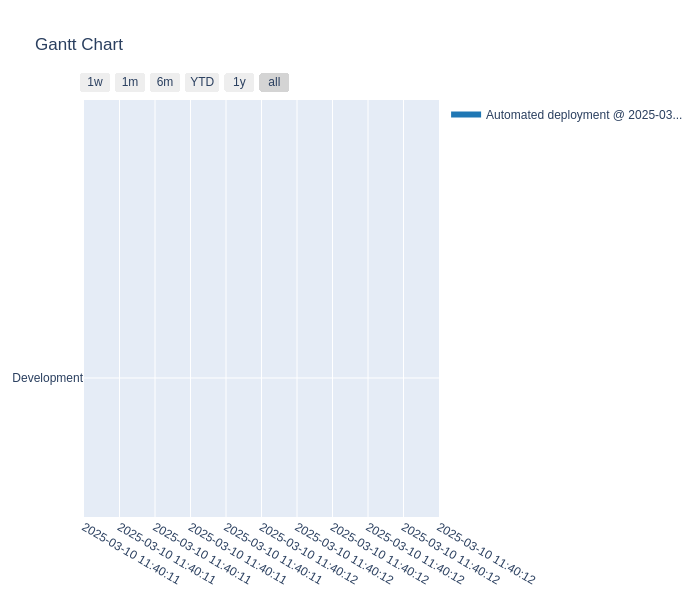
### Commit Activity Heatmap
This heatmap shows the distribution of commits over the past year:
![Commit Heatmap]()
### Contributor Network
This network diagram shows how different contributors interact:
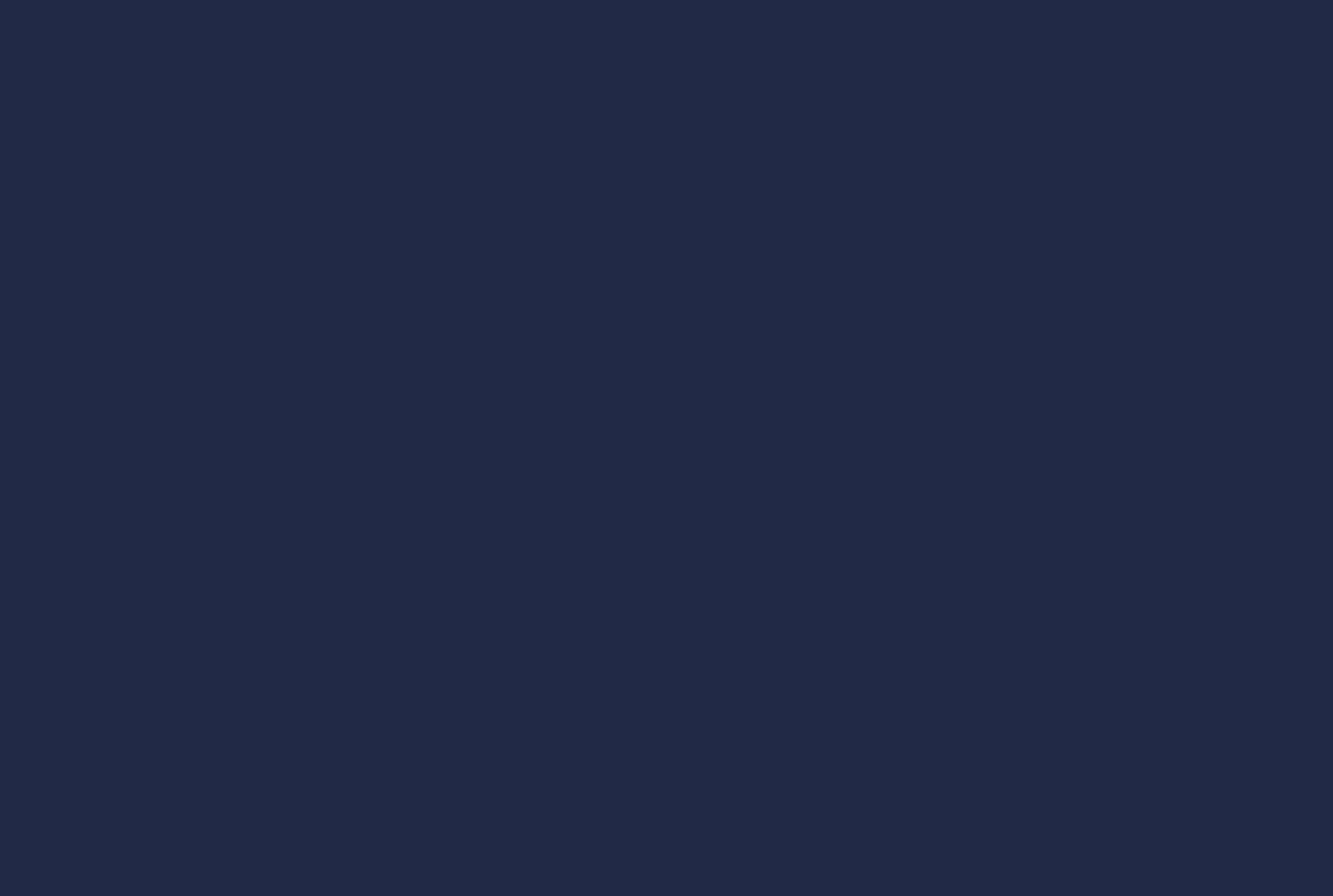
### Commit Activity Patterns
This chart shows when commits typically happen:
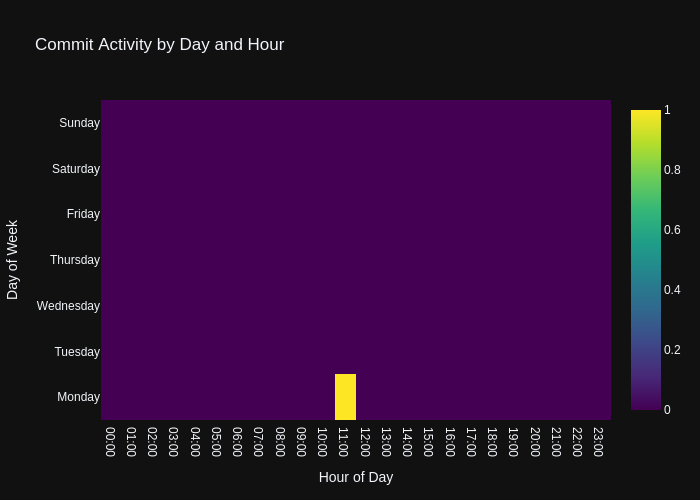
### Code Frequency
This chart shows the frequency of code changes over time:
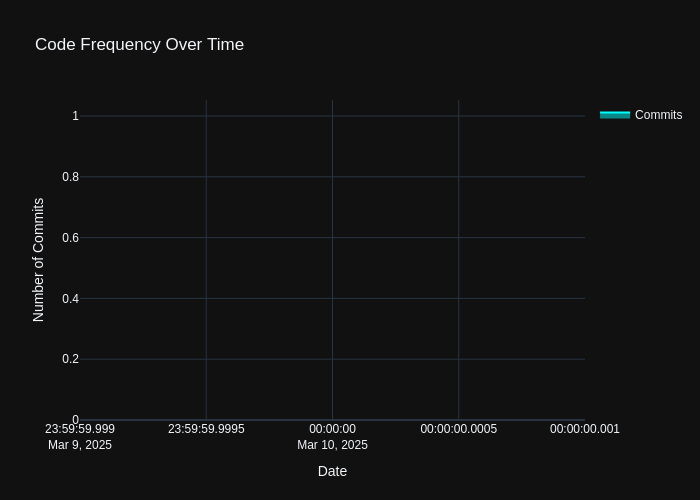
* Repository URL: [https://github.com/wanghaisheng/AI-automatically-generates-novels](https://github.com/wanghaisheng/AI-automatically-generates-novels)
* Stars: **0**
* Forks: **0**
编辑整理: Heisenberg 更新日期:2025 年 3 月 17 日